Machine Learning for Malware Detection: Revolutionizing IT Security
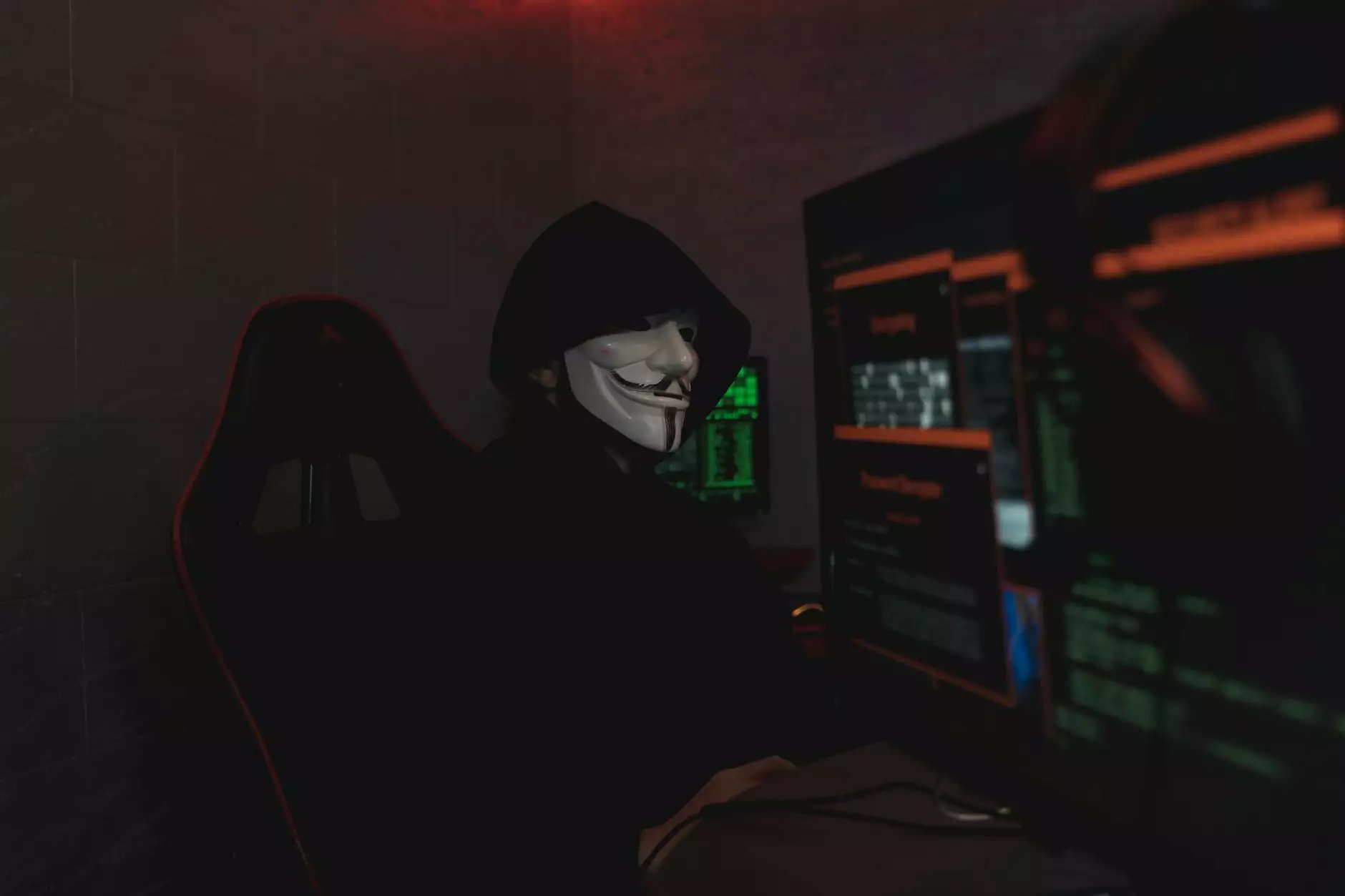
In today’s digital age, the increasing prevalence of malware poses a significant threat to organizations across the globe. The evolution of these malicious software variants demands a more sophisticated approach to cybersecurity. Machine learning for malware detection has emerged as a powerful tool in the arsenal of IT security professionals. This article aims to detail the myriad ways in which machine learning enhances malware detection, providing unrivaled protection to businesses like Spambrella, specializing in IT Services and Security Systems.
Understanding Malware: A Growing Threat
Malware, short for malicious software, encompasses various types of intrusive software designed to exploit and damage devices and networks. In the context of IT security, it is critical to recognize the different forms of malware, including:
- Viruses: Programs that replicate themselves by attaching to other files.
- Worms: Standalone software that can self-replicate and spread across networks.
- Trojans: Malicious programs disguised as legitimate software.
- Ransomware: Software that encrypts files and demands a ransom for access.
- Spyware: Software that secretly monitors user actions and collects information.
Each type of malware employs different techniques to infiltrate systems, making traditional detection methods inefficient. This is where machine learning for malware detection comes into play, offering advanced capabilities to analyze and mitigate these threats effectively.
The Role of Machine Learning in Cybersecurity
Machine learning, a subset of artificial intelligence, involves the use of algorithms and statistical models to enable systems to improve their performance on a specific task through experience. In the context of malware detection, machine learning techniques can analyze massive datasets to identify patterns, detect anomalies, and predict potential threats without human intervention.
Key Benefits of Machine Learning for Malware Detection
Integrating machine learning into malware detection frameworks provides several benefits, including:
- Increased Accuracy: Machine learning algorithms can reduce false positives and false negatives, allowing security systems to detect real threats more accurately.
- Real-time Processing: With the ability to process data in real-time, machine learning systems can identify and respond to malware threats instantaneously.
- Adaptive Learning: Machine learning models can continuously evolve by learning from new data, improving their detection capabilities over time.
- Automated Threat Intelligence: Automated systems can collect, analyze, and disseminate threat intelligence efficiently, ensuring timely updates to security measures.
How Machine Learning Detects Malware
To understand how machine learning enhances malware detection, one must delve into the algorithms and techniques used in this field. Some of the fundamental methods include:
1. Supervised Learning
In supervised learning, models are trained on a labeled dataset, where the algorithm learns to associate specific input patterns with their corresponding outputs (malicious or benign). Techniques such as Decision Trees, Support Vector Machines (SVM), and Neural Networks are commonly employed in this category.
2. Unsupervised Learning
Unsupervised learning does not rely on labeled data. Instead, it identifies patterns and structures within the data itself. Clustering algorithms such as K-means and Hierarchical Clustering can detect hidden threats by analyzing features of previously unseen malware.
3. Deep Learning
Deep learning, a more advanced subset of machine learning, utilizes artificial neural networks with multiple layers. This approach excels in feature extraction and is particularly effective at detecting complex malware variants by mimicking the way humans learn.
Implementing Machine Learning in Malware Detection Systems
Organizations seeking to leverage machine learning for malware detection need to integrate these technologies into their cybersecurity infrastructure thoughtfully. This process generally includes the following steps:
1. Data Collection
Gathering diverse datasets is crucial. Data sources can include network traffic logs, file attributes, process behaviors, and more. The quality and quantity of data directly influence the effectiveness of the machine learning models.
2. Feature Selection
Choosing the right features is essential for building efficient models. Relevant features may comprise:
- File size and type
- API calls made by a program
- Network behavior patterns
- User access logs
3. Model Training
After preparing the data, the next step involves training the machine learning models using the curated datasets. This phase may require experimentation with different algorithms to identify which performs best regarding detection accuracy and speed.
4. Testing and Validation
Once trained, it is vital to validate the models using independent datasets to ensure they perform well in real-world scenarios. Metrics such as accuracy, precision, recall, and F1 score should be analyzed during this stage.
5. Deployment
After successful testing and validation, the models can be deployed within an organization’s IT infrastructure. Continuous monitoring ensures that the models remain effective against evolving threats.
Challenges Facing Machine Learning in Malware Detection
Despite its numerous advantages, the deployment of machine learning for malware detection does face challenges:
1. Evasion Techniques
Malware developers are constantly updating their tactics to evade detection. Techniques such as obfuscation can render models ineffective if they are not adequately trained to deal with these variations.
2. Data Privacy and Ethics
The collection and usage of data for training machine learning models raise concerns about privacy and ethical considerations. Organizations must ensure that they adhere to regulations and best practices.
3. Resource Intensive
Machine learning systems often require substantial computational resources and skilled personnel to develop and maintain. This need can be a barrier for small businesses, though cloud-based solutions are emerging to alleviate this issue.
The Future of Machine Learning in Malware Detection
The evolution of machine learning for malware detection is promising, with ongoing advancements in technology paving the way for superior security measures. Future trends include:
- Integration with AI: Further integrating AI technologies can lead to more robust security frameworks, capable of preemptively identifying and neutralizing threats.
- Collaborative Intelligence: Building a collaborative network where organizations share threat intelligence will enhance detection capabilities across the board.
- Enhanced User Education: As systems become more complex, educating users on recognizing risks will complement technical defenses, creating a holistic security approach.
Conclusion
As businesses rely increasingly on technology, the importance of effective malware detection cannot be overstated. Machine learning for malware detection provides a cutting-edge solution that enhances traditional cybersecurity methods, ensuring organizations can defend against an ever-evolving threat landscape. Companies like Spambrella exemplify how embracing these technologies can reinforce their mission to provide comprehensive IT services and security systems. By investing in machine learning solutions, businesses can not only protect their assets but also gain a competitive edge in today’s fast-paced digital environment.